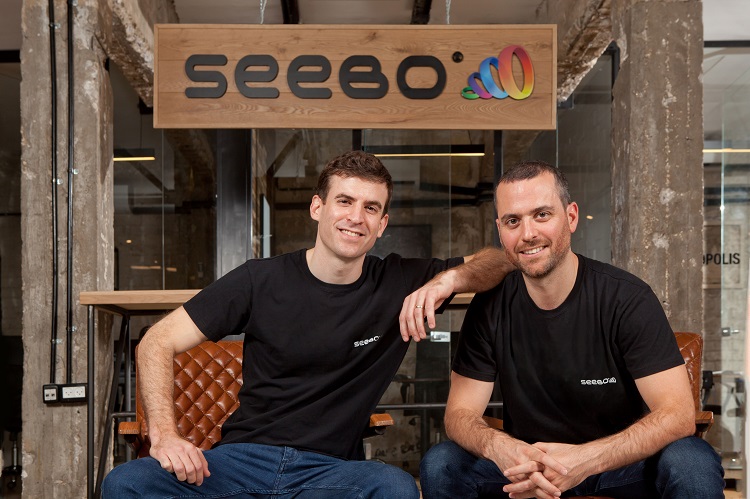
In production, waste comes in many shapes and sizes. For some businesses, it could be ‘reject’ products that don’t fit the desired weight, size, or shape. For others, it could be a question of quality – such as colour or moisture variances – or raw materials variability.
Whatever the form of the waste, production losses are ‘very important challenges to deal with’, according to Seebo co-founder and CEO Lior Akavia.
The start-up was born out of a desire to cut waste in production lines, both in prediction and prevention. “We realised that medium and large food manufacturers usually lose, on average, between dozens to hundreds of millions of dollars every year due to waste issues,” he told FoodNavigator.
The AI-based software technology, which integrates with existing production sensors, is also designed to better companies’ bottom lines. “Manufacturers that will be able to successfully reduce waste will become more competitive in the marketplace.
“These manufacturers can save millions of dollars a year by effectively reducing production waste.”
How does it work?
While Seebo works across a number of industries, the majority of its customers work in food. Big names on its books include Nestlé, Barilla, and Mondelēz.
By onboarding the start-up’s AI-based software, Seebo gathers data from multiple sources, including raw materials, productions lines, and quality results, before kickstarting the algorithm process.
“Our algorithms are very focused on the topology of the production line, on the interdependences between the different assets and the different steps of the production process,” the CEO explained.
Ultimately, Akavia said, the software allows production teams to answer three main questions.
Firstly: why do process inefficiencies happen? Seebo responds to this particular question through the use of automated root cause analysis. Secondly: how can we prevent process inefficiencies? The start-up provides predictive recommendations to businesses to solve this problem. And thirdly: when should we act in order to prevent such inefficiencies? Here, Seebo relies on proactive alerts to warn the production team before a waste event occurs.
Where a root cause for a waste event is identified, production teams can change the recipe or alter a set point in order to prevent ‘many waste occurrences’, we were told.
The AI element does of course present both opportunities and challenges. Seebo’s ‘AI engine’ itself is not intuitive, nor accessible to those outside of the data science sphere. Translation, therefore, between the hard data findings and production teams is key.
Akavia described it as a ‘two-way dialogue’: “We want the user to get intuitive and actionable insights on one hand, and on the other, we want the AI to understand the feedback from the user…
“The user defines the exact problems it wants to avoid – for example avoiding waste due to underweight – and the AI should understand that.”
The CEO said Seebo’s ‘very visual user interface’ is a ‘strong tool’ that helps make it intuitive and ‘easy to use’.
COVID boosts demand for complex lines
Seebo’s offering is most effective where production processes are ‘relatively complex’. This means that the technology is ‘less relevant’ for simpler set-ups such as sugar or beverage production lines.
“One important criteria is the complexity [of the production]. In general, when it is more complex, we are generating more value for our clients,” Akavia explained. Ultimately, the more complex a production process, the more opportunities for waste to occur. “It’s also more challenging for the human brain to digest so many parameters that are constantly changing,” added the CEO.
A wide range of food production lines are complex, including those with high ingredient counts such as chocolate, bakery, and confectionery.
Another criteria is how automated or manual the process is. If a process is completely manual, Seebo will not have the data required to drive its software. “All our clients have either semi-automated or completely automated productions.”
For businesses that fit the production criteria, Akavia says Seebo can help reduce losses, and as a result, simultaneously increase capacity. “In general, the ability to reduce losses is a very strong motivation, because it contributes directly to the net profit of the company.
“It also increases customer satisfaction, because when you can do things in higher quality, eventually you will get fewer customer complaints.”
The onset of the coronavirus pandemic has accelerated this demand. Companies are not only looking to cut losses, increase efficiency, and improve their financials, but they are also under pressure to maintain supply in the face of unprecedented spikes in demand. “For quite a few of our manufacturers, demand for their food products increased in dozens of percentages after COVID-19 started,” said the CEO.
Manufacturers were left with two choices: to either build new production lines to meet demand – but ‘this takes years’ and ‘huge investments’, said Akavia – or optimise their processes, which can ‘quickly translate into increased capacity’.
Seebo completes $9m funding round
Moving forward, Seebo wants to expand its customer base and ‘help more manufacturers reduce waste’.
A recently closed funding round of $9m (€7.7m) will help the start-up do just that. The round was led by Ofek Ventures, with the participation of Vertex Ventures, and existing investors Viola Ventures and TYP Capital. To date, Seebo has raised a total of $31m.
Ofek Ventures partner Itay Rand said Seebo has ‘cemented’ itself in the fields of predictive quality and industrial AI. “Manufacturers today understand that in order to compete successfully they must adopt effective process optimisation capabilities, and there is a clear recognition that industrial AI and a data-driven approach is fundamental to achieving that goal.”
Seebo will also use the money to ‘continuously improve’ its AI engine, we were told. “We believe that from the technological perspective, we have the best AI solution in the world to deal with production waste problems. But we want to solidify that position and we want to maintain this position. To do so…we need to continuously invest in research and development and provide new features and new generations of our technology.”